Reactive Flows and Multiphysics Simulations
Multiphysics simulations involve the computational modeling of systems where multiple physical phenomena, such as fluid dynamics, chemical reactions, heat transfer, or electromagnetics, interact simultaneously. These simulations enable to analyze complex, coupled behaviors in engineered systems or natural processes, leading to optimized designs and deeper scientific insights.
Our research spans a variety of fields, including combustion, multiphase flows, and aerosols. We study laminar and turbulent hydrogen flames, along with other emerging carbon-neutral fuels, to support the transition toward a clean and sustainable energy future. Additionally, we investigate the formation and transport of small particles in fluids, aiming to reduce toxic particulate emissions from airplanes and industrial processes and to optimize the synthesis of valuable nano- and microparticles.
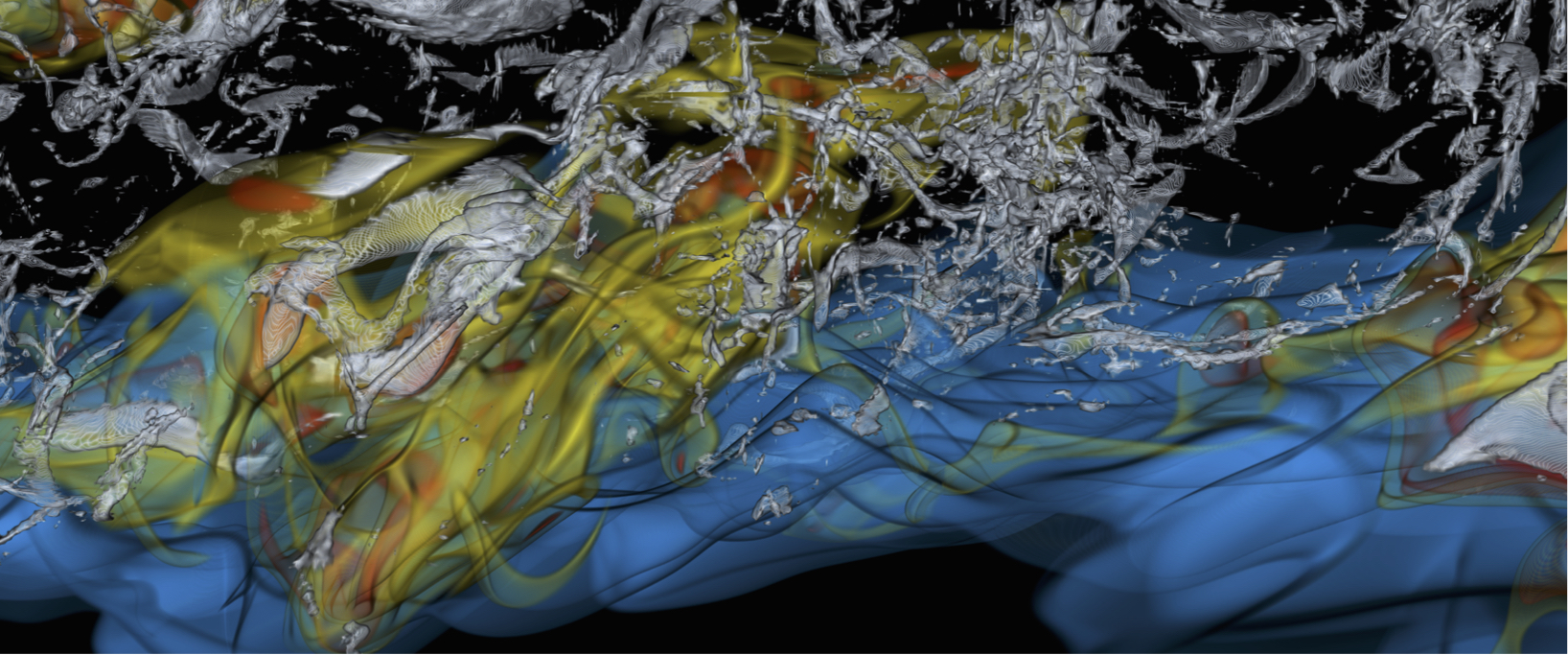
Visualisation of particulate (gray) in a reactive flow simulated with Direct Numerical Simulation
Aerodynamics and Turbulence
The Last Unsolved Problem of Classical Physics (Richard Feynman)
I am an old man now, and when I die and go to Heaven, there are two matters on which I hope for enlightenment. One is quantum electrodynamics, and the other is the turbulent motion of fluids. And about the former, I am rather optimistic. (Horace Lamb)
Turbulence is the chaotic and unpredictable motion of a fluid, such as the flow over an airplane wing, the gusty air on a windy day, or the plume of a rocket. It is a fascinating phenomenon that blends chaos and order, shaping our natural world in profound ways—from the patterns of clouds in the sky to the efficiency of engines and the behavior of ocean currents. Despite its ubiquitous presence in everyday life, turbulence remains one of the most complex and unsolved mysteries in physics, continually challenging our understanding of nature's fundamental laws.
In our group, we study the fundamentals of turbulence and its interactions with other phenomena. Our goal is to unravel the intricate dynamics of turbulent flows, discover universal laws that apply to any turbulence problem, and develop simplified models that can be used in industrial design.
Transition from Kelvin-Helmholtz vortices to turbulence in a mixing layer
Machine Learning and Generative AI
A poem about turbulence and ML
by chatGPT (2023)
In the midst of swirling winds and storms,
A world of chaos that never conforms,
The science of turbulence has long been sought,
To understand its patterns, and how it can be fought.
But with the rise of machine learning's might,
We have a tool to delve into the fray,
To analyse the data and the flight,
And uncover insights in a new way.
With algorithms trained on vast amounts of data,
Patterns emerge that we may not have seen before,
Hidden relationships and connections are revealed,
And insights into turbulence are unsealed.
Turbulence, a mystery yet to unfold
A never-ending story, yet to be told
With every discovery, new and old
Turbulence, forever, it will hold.
Scientific Machine Learning and AI for Science are revolutionising numerous fields, from image and speech recognition to quantum computing. In recent years, these technologies have made significant inroads into various scientific domains, often leading to more efficient solution methods. Within the realm of fluid mechanics, there have been remarkable advancements in applications such as turbulence and aerodynamics, weather forecasting, climate science, and, more broadly, engineering design and virtual prototyping.
In our group, we focus on developing and applying innovative machine learning and generative AI techniques to address complex challenges in fluid mechanics. Our work includes modelling turbulence and combustion, the super resolution of complex fluid flow simulations, and identifying and predicting extreme or catastrophic events. Additionally, we leverage generative AI to compress extremely large datasets and employ interpretable machine learning to uncover the intricate dynamics of multiscale features that are ubiquitous in turbulent flows.
Large-scale simulation data used to train Neural Networks
High Performace Computing and Data Analytics
Multiphysics problems span vast ranges of length and time scales, from nanometers to kilometres and from nanoseconds to minutes. Tackling these challenges requires cutting-edge simulation tools that leverage highly efficient computational methods and can operate on the world’s most powerful supercomputers. Additionally, advanced AI methods demand significant computational resources, often relying on specialised hardware such as GPUs. Beyond performance, sustainability is paramount—making computational efficiency a key priority to reduce energy consumption.
In our group, we develop highly accurate and efficient numerical methods with a dual focus: expanding the scale and range of simulation conditions while simultaneously minimising the energy consumption of these computational tools.
These large-scale simulations produce enormous amounts of data, often exceeding hundreds of terabytes. Such data are invaluable for investigating the underlying physics and training large neural network models but require careful handling. We develop advanced techniques for data analysis, such as the Dissipation Element concept and machine learning-based analysis tools, along with data compression methods, to manage this data in an agile and efficient manner.
A dissipation element in a turbulent flow